Artificial intelligence (AI) has become a driving force for innovation across various sectors. The technology’s versatile nature makes it adaptable to various applications, from predicting consumer behavior to facilitating autonomous vehicles and now, in the domain of program evaluation. However, to fully appreciate the potential of AI in program evaluation, we first need to understand what AI truly is.
What is AI?
AI, in a nutshell, is a branch of computer science dedicated to creating intelligent machines capable of performing tasks that would typically require human intelligence. These tasks include learning, reasoning, problem-solving, perception, language understanding, and creativity.
AI can be categorized into two main types: narrow AI, designed to perform a narrow task (like voice recognition), and general AI, which can perform any intellectual task that a human being can.
The strength of AI is that it is not a singular method or technology. Instead, it is a cluster of methods and techniques, all working in unison to mimic and augment human intelligence. These methods range from traditional Machine Learning (ML) techniques to advanced Deep Learning (DL) models and Generative AI models.
Range of Methods in AI
AI encompasses various methods, each with different capabilities and applications.
- Machine Learning (ML): ML, a subset of AI, focuses on developing algorithms that allow computers to learn from and make data-based decisions. Techniques like regression analysis, decision trees, and clustering are part of ML.
- Deep Learning (DL): DL is an advanced subset of ML. It’s designed to mimic the human brain’s workings through artificial neural networks, enabling machines to learn from vast amounts of unstructured data.
- Natural Language Processing (NLP): NLP is the branch of AI that helps computers understand, interpret, and generate human language. I love, love, love NLP. It’s not a stretch to say it’s been transformative to me in how I think about evaluation design, but that’s a topic for another blog.
- Computer Vision: This field helps computers “see,” process, and understand digital images or videos like human vision.
- Reinforcement Learning (RL): RL is a type of machine learning where an agent learns to behave in an environment by performing actions that are either rewarded or penalized.
AI in Program Evaluation
In the context of program evaluation, AI can bring significant advancements. Traditionally, program evaluation involves data collection, analysis, and interpretation to assess the performance and effectiveness of a program. AI can contribute to each of these stages.
- Data Collection: AI, particularly NLP and computer vision, can automate and enhance the process of data collection, handling complex and unstructured data like text, images, and real-time interactions.
- Data Analysis: ML and DL techniques can significantly improve the speed and accuracy of data analysis. They can uncover patterns and relationships within the data that may be impossible for humans to detect. This is the stuff we love. We’ve been implementing NLP with text-based data for a while now (both with our program evaluation data and over at Layrr.ai for scientific data.
- Interpretation and Reporting: AI can assist in interpreting results and creating intuitive reports. NLP can generate clear, understandable narratives from complex data sets.
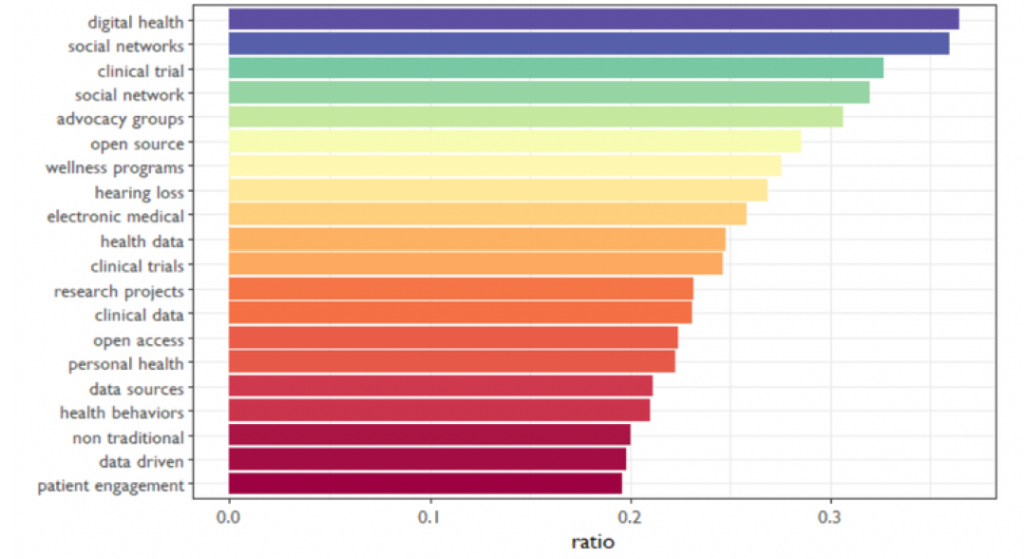
The Future: Generative AI in Program Evaluation
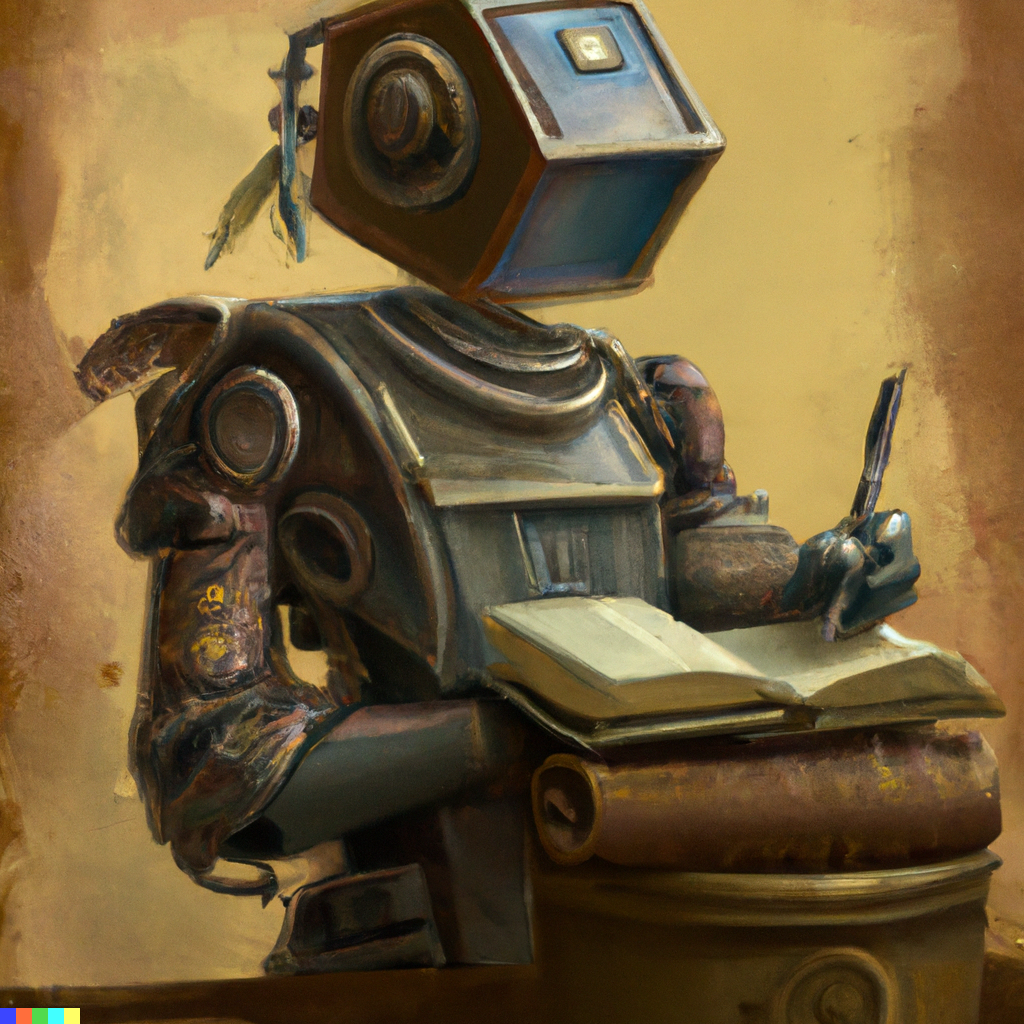
Generative AI, a rising field in AI, can create content from scratch, whether a piece of music, an image, or even a block of text. It uses advanced models like Generative Adversarial Networks (GANs) and Transformers (like GPT-4) to produce novel outputs.
So, how can generative AI contribute to program evaluation?
- Generating Simulation Data: Generative AI can create realistic, synthetic data sets that can help in testing different scenarios in program evaluation, helping evaluators to predict and prepare for a range of potential outcomes.
- Enhancing Decision-making: Generative AI can facilitate more nuanced decision-making by creating detailed predictive models of potential program outcomes.
- Improved Reporting: Generative AI can potentially automate the generation of comprehensive evaluation reports, including text, charts, and even presentations, simplifying the communication of evaluation findings.
To sum it up, AI provides an array of techniques that can revolutionize program evaluation. From automating data collection and analysis to enhancing interpretation and reporting, AI brings unprecedented efficiency and accuracy. The future of AI, particularly in the form of generative models, promises even greater advancements, enabling evaluators to generate insightful predictions, facilitate decision-making, and communicate their findings more effectively.
Using AI to monitor research
Head on over to Layrr to see how we use AI to monitor and synthesize publications. Every week, for free, we send out This Week in Science and This Week in Public Health. Sign up here!